
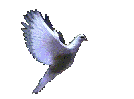
|
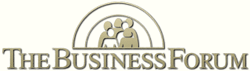
"It is impossible for ideas to
compete in the marketplace if no forum for
their presentation is provided or available."
Thomas Mann, 1896
The Business Forum
Journal
The New
Paradigm in Reducing Employer Health Care Costs
By Dennis
Cannelis
Current Health Care Cost Climate
Health care costs continue to
rise. Total health care costs per employee are expected to rise above
$10k in 2010. Employers face an average year over year increase of 7%.
21% of the workforce suffers
from a chronic condition, accounting for fully 75% of all medical costs.
Health risks such as blood pressure and high cholesterol account for 25%
of total health care costs.
However, 70% of health care
claims have shown to be preventable. The key opportunity to reducing
costs today is to focus on wellness, that is, to target the demand side
of healthcare � identify behavioral and lifestyle factors and manage or
improve risk factors to prevent more costly ongoing treatments.
Corporate wellness programs can
decrease employee absenteeism by 26% and can decrease health care costs
by 27%.
The key to these programs is a
comprehensive approach where participation of the employee through
incentive design is the key to a successful health management program.
The AIM
Approach
In addition a program
consisting of Assessment (through health risk assessments, biometric
screenings, and Claims Analytics), Interaction (through outreach
programs measuring health risk scores and achievements, and clinical
compliance programs), and Measurement (tangible results of health
improvement) utilizing incentives to drive participation is a key to
successfully reducing costs.
In addition, the program would
have to rely on analytics for population metrics. Information
Technology is being utilized to promote and manage innovative strategies
and programs that make use of proactive metrics to reduce the cost of
healthcare delivery.
Another key
factor in wellness programs is Analytics
In fact, self insured employer
groups, provider organizations, and health insurance payers that focus
on state and federally reimbursed insurance programs all are keenly
focused on analytics that can identify the potential for risk of illness
and disease in population management profiles through identification of
user defined metrics that can be defined with the help of health care
delivery professionals and insurance organizations. The patient profile
is a dynamic record - a snapshot of the employee�s history, current
health status, compliance, and a record of specific test results. This
information will enhance the ability to determine an individual�s risk
for other problems. Information will be collected automatically
wherever possible via the Engagement Analytics engine to create a
stratified layer of comprehensive information from disparate sources:
The differentiator in this
approach is that the aggregation of this information creates a member
profile that proactively determines the potential for risk rather than
traditional disease management analytics retroactively searching for
information that is either unavailable, incorrect, or lagging. This is
hit or miss at best and is one of the problems with typical disease
management programs.
Instead, this is a central
repository that incorporates all of the different aspects of a case-
history could be updated automatically when the record is first
implemented with a retrospective claims analysis using population
screening metrics. History is an important consideration that impacts a
person's risk for disease-yet, it is typically 'collected' via HRA or
some sort of voluntary admission by the member/patient. That is one way
to do it, but there is also a wealth of information in the previous
years� (if available) claims data--if there are claims with diagnosis
that indicate chest pain or cancer or anything that is either a serious
symptom, complication or disease -chronic or acute, then it is
considered history. This eliminates the need to try to figure out how
to build on those 'English' statements that can not be mapped to ICD-9
and/or CPT-4 codes. Risk can be identified for multiple diseases if a
search is performed on the member's history for smoking, high blood
pressure, high cholesterol, low LDL cholesterol, diabetes,
cardiovascular conditions and obesity. If the history is positive for
any of these things, that information is appended to the member profile
- it remains there as a factor that determines that member's status.
Analytics for Diabetes Example:
A member being tested for glucose, who has metrics for history, risk,
age, etc., would serve as a trigger to get those results. The
combination of data that is collected will reveal co- morbid conditions
automatically.
One example is the glucose
tolerance test, which is used to monitor diabetics, screen for
pre-diabetes due to either diabetics, screen for pre-diabetes due to
either risk factors or symptoms, and is also a preventive measure for
everyone over 45. While the reason for the test may not be known
initially, it should be a pro-active �red flag�, since it is the
single most common way that pre-diabetes and diabetes are first
diagnosed. This presents an opportunity for a high value intervention
since someone with impaired glucose is likely to develop diabetes II
within two years without intervention. However, in order achieve the
ultimate goal of pre-diabetes intervention; results of the test would be
required. There are a couple of different scenarios that are possible:
Example: The test reveals a
high glucose level and results in a diagnosis of pre-diabetic or
diabetic, in which case the system would pick that up on a subsequent
screening/surveillance, IF the patient returns to the doctor. Since the
lab is not the one who does the diagnostic follow up and the lab is not
the entity that communicates with the payer except to send a bill with
the service rendered - results will not be known, or even why the test
was ordered until if or when a subsequent claim is received for that
diagnosis. This could be months later, depending on several factors
including claims lag and patient's compliance with the outcome, or, the
patient is complying with preventive measures and does not require
follow up. There will not be a clear difference between healthy
preventive, good outcome and non-compliance, risky outcome without
further intervention.
Combined with the member's
demographics, the data that is accumulated in the file determines a
member's health status.
Here are other possible
scenarios for engagement analytics searches possible based on metrics
accumulated:
�Anyone ≥45 and BMI= ≥26, or,
anyone ≤45 and BMI= ≥ 26 and, has one or more risk factors [add dx.xx],
then get tested. Then, if IFT results =100-125, or IGT results =
140-199, then re � test again in 1-2 years�.
Conclusion
The key factors to a successful
wellness program are : providing a way to identify potential problems
through analytics and other forms of assessment, to enroll those
employees in employer a defined wellness outreach programs, incent their
behavior to participate, and to use analytics to measure these results.
Dennis Cannelis
is a Fellow of The Business Forum Institute and
is recognized as a serial
entrepreneur specializing in healthcare Information Technology.
He currently serves as Vice President of Information Technology
for Community Health Plan of Washington. Dennis has more than
twenty five years experience in Information Technology, Software
Development in the fields of Healthcare Services, Professional
Services, Investment Banking, International Markets, and
Manufacturing; and has served in several roles as either CEO,
Managing Partner, Senior Vice President and Management
Consultant. Dennis holds a B.A. from New York University
and a certification in Information Systems Management from
Program Systems Institute.
Visit the Authors Web Site
~
http://www.cbshc.com/
To Contact
the Author:
~ Click Here
Return to
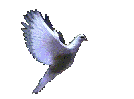
The Business
Forum Journal
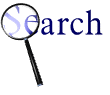 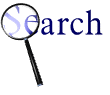 Search
Our Site
Search the ENTIRE Business
Forum site. Search includes the Business Forum Library, The Business Forum Journal and the Calendar Pages.
Editorial Policy: Nothing you read in
The Business Forum Journal
should ever be construed to
be the opinion of, statements condoned by, or advice
from, The Business Forum, its staff, workers, officers, members, directors, sponsors or shareholders. We pass no opinion whatsoever on the content
of what we publish, nor do we accept any responsibility for the claims, or
any of the statements made, within anything published herein. We merely
aim to provide an academic forum and an information sourcing vehicle for
the benefit of the business and the academic communities of the Pacific States of America
and the World.
Therefore, readers must always determine for themselves where the statistics, comments, statements and
advice that are published herein are gained from and act, or not act, upon such entirely and always at their own risk. We
accept absolutely no liability whatsoever, nor take any responsibility for
what anyone does, or does not do, based upon what is published herein, or
information gained through the use of links to other web sites included
herein. Please refer to our:
legal
disclaimer
Home
Calendar The Business Forum Journal
Features
Concept
History
Library
Formats
Guest Testimonials
Client Testimonials
Search
News Wire
Why Sponsor
Tell-A-Friend
Join
Experts
Contact The Business Forum
The Business Forum
Beverly Hills, California United States of America
Email:
[email protected]
Graphics by
DawsonDesign
Webmaster:
bruceclay.com
� Copyright The Business Forum Institute 1982 - 2011 All rights reserved.

|
|
|
|